The Role of Big Data in Fintech - Driving Insights and Decisions

1. What is Big Data in Fintech?
Big data refers to the vast volume of structured and unstructured data generated by digital activities. In fintech, it includes data from:
- Transactions: Payments, deposits, withdrawals, and transfers.
- Customer Behavior: Browsing patterns, spending habits, and preferences.
- Market Trends: Stock movements, currency fluctuations, and economic indicators.
- Risk Assessments: Fraud patterns, credit scores, and compliance metrics.
Key Technologies Driving Big Data Analytics:
- Artificial Intelligence (AI): Processes large datasets to detect patterns and trends.
- Machine Learning (ML): Learns from data to make predictions and automate tasks.
- Cloud Computing: Enables scalable data storage and processing.
- Blockchain: Ensures data integrity and security.
- Natural Language Processing (NLP): Analyzes unstructured data, such as text and voice inputs.
2. Applications of Big Data in Fintech
1. Personalized Customer Experiences:
- Example: AI-powered robo-advisors offer customized investment strategies.
- Impact: Increases customer engagement and satisfaction through tailored recommendations.
2. Fraud Detection and Prevention:
- Example: Machine learning algorithms analyze transaction patterns to detect anomalies.
- Impact: Enhances security and reduces false positives in fraud prevention systems.
3. Credit Scoring and Lending Decisions:
- Example: Alternative credit scoring models assess risk based on behavioral data.
- Impact: Expands access to credit for underserved markets.
4. Predictive Analytics for Investment Strategies:
- Example: Hedge funds use big data to identify profitable market trends.
- Impact: Enables smarter, data-driven investment decisions.
5. Risk Management:
- Example: Real-time monitoring tools assess compliance risks and identify vulnerabilities.
- Impact: Mitigates threats before they escalate, ensuring regulatory adherence.
6. Customer Support and Chatbots:
- Example: NLP-powered chatbots provide instant assistance and issue resolution.
- Impact: Reduces operational costs and improves service quality.
3. Benefits of Big Data Analytics in Fintech
1. Improved Decision-Making:
- Data-driven insights enable faster, more accurate decisions.
2. Enhanced Efficiency and Automation:
- Reduces manual processes through AI and machine learning.
3. Better Risk Assessment:
- Predicts market trends and identifies vulnerabilities to prevent losses.
4. Customer-Centric Strategies:
- Personalizes offerings, improving customer loyalty and retention.
5. Competitive Advantage:
- Provides actionable insights for product innovation and market positioning.
4. Challenges in Leveraging Big Data
1. Data Privacy and Security:
- Issue: Handling sensitive financial data raises privacy concerns.
- Solution: Implement encryption, tokenization, and compliance frameworks like GDPR.
2. Data Quality and Accuracy:
- Issue: Inconsistent or incomplete data may lead to faulty insights.
- Solution: Use data cleansing tools and regular audits to ensure accuracy.
3. Scalability Issues:
- Issue: Growing datasets require robust infrastructure.
- Solution: Adopt cloud-based platforms for scalable storage and processing.
4. Compliance Complexity:
- Issue: Navigating regulations across regions can be challenging.
- Solution: Employ RegTech solutions to automate compliance monitoring.
5. Integration with Legacy Systems:
- Issue: Older systems may lack compatibility with advanced analytics tools.
- Solution: Use APIs to enable seamless integration and migration.
5. Strategies for Implementing Big Data Analytics
Step 1: Define Business Goals
- Identify specific challenges or opportunities that big data can address.
Step 2: Invest in Data Infrastructure
- Deploy cloud-based storage solutions for scalability and cost-efficiency.
Step 3: Leverage AI and Machine Learning Tools
- Train algorithms to predict trends, detect fraud, and automate processes.
Step 4: Focus on Data Security and Privacy
- Implement encryption, access controls, and compliance measures.
Step 5: Build a Data-Driven Culture
- Train employees to use analytics tools effectively and encourage data-driven decision-making.
Step 6: Test, Monitor, and Optimize
- Continuously analyze performance metrics to refine strategies and improve outcomes.
6. Future Trends in Big Data for Fintech
1. Predictive Behavioral Analytics:
- AI models forecasting spending habits and market shifts.
2. Real-Time Data Processing:
- Instant analysis for fraud detection and trading strategies.
3. Hyper-Personalization:
- AI-driven insights offering dynamic, customized experiences.
4. Blockchain Integration:
- Secure and transparent data sharing across financial networks.
5. IoT and Big Data Fusion:
- Data collection from smart devices to monitor transactions and behavior.
6. Explainable AI (XAI):
- Ensuring transparency in AI decisions to improve regulatory trust.
Big Data is Reshaping Fintech Innovation
Big data analytics has become a vital tool for fintech companies, enabling smarter decision-making, enhanced customer experiences, and robust security measures. By leveraging AI, machine learning, and cloud technologies, fintech firms can unlock actionable insights, optimize operations, and stay competitive.
However, businesses must address challenges like data privacy and integration to fully harness big data's potential. With the right strategies, fintech companies can transform data into a strategic asset, paving the way for sustainable growth and innovation.
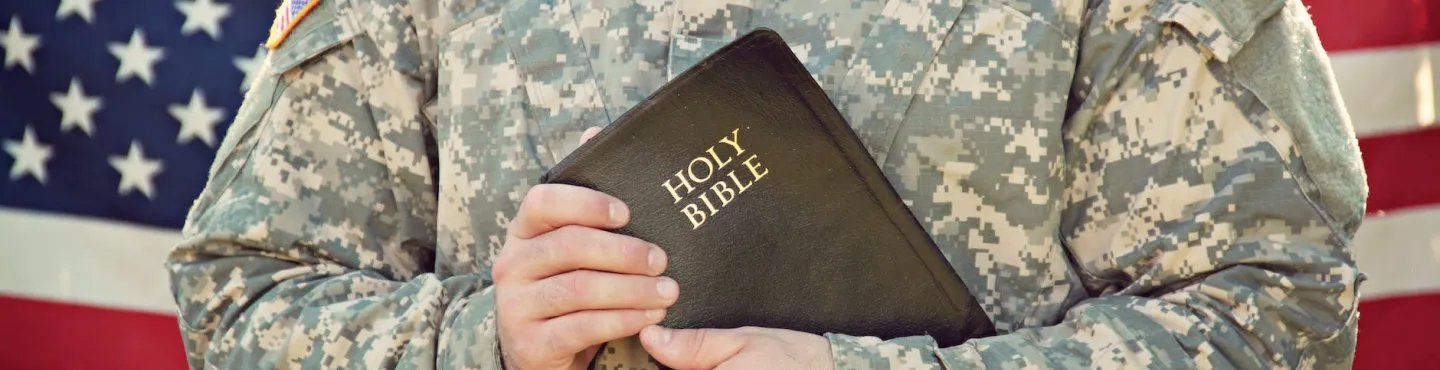