AI and Machine Learning in Risk Management - Transforming Fintech Security

1. The Role of AI and ML in Risk Management
AI and ML leverage vast datasets to identify patterns, predict outcomes, and automate responses. Unlike traditional risk models that rely on static rules, AI adapts to new threats and trends in real time.
Key Capabilities:
- Predictive Analytics: Forecasts risks based on historical data.
- Anomaly Detection: Identifies deviations from normal patterns.
- Natural Language Processing (NLP): Analyzes unstructured data like emails and social media for risk signals.
- Automation: Reduces manual intervention by triggering automated workflows.
2. Applications of AI and ML in Fintech Risk Management
1. Fraud Detection and Prevention:
- Use Case: ML algorithms analyze transaction patterns to detect anomalies and flag suspicious activities.
- Impact: Reduces false positives and improves fraud prevention accuracy.
2. Credit Scoring and Risk Assessment:
- Use Case: AI models assess borrower risk based on behavioral data, alternative credit scores, and real-time inputs.
- Impact: Expands access to credit for underserved markets while lowering default risks.
3. Anti-Money Laundering (AML) Compliance:
- Use Case: AI detects complex money-laundering schemes by analyzing transactional flows and behaviors.
- Impact: Simplifies compliance with AML and KYC regulations.
4. Cybersecurity and Threat Intelligence:
- Use Case: AI monitors network activity to detect phishing, ransomware, and unauthorized access attempts.
- Impact: Reduces response times and improves threat detection capabilities.
5. Portfolio and Investment Risk Management:
- Use Case: ML algorithms predict market trends and assess portfolio vulnerabilities.
- Impact: Enables dynamic risk-adjusted investment strategies.
6. Regulatory Reporting and Monitoring:
- Use Case: AI automates compliance reporting, ensuring accuracy and efficiency.
- Impact: Lowers compliance costs and reduces human error.
3. Benefits of AI and ML in Risk Management
1. Proactive Risk Mitigation:
- AI predicts threats before they materialize, enabling preemptive actions.
2. Real-Time Monitoring:
- ML systems continuously analyze data streams for anomalies, providing instant alerts.
3. Cost Reduction:
- Automates repetitive tasks, lowering operational costs and resource requirements.
4. Scalability:
- Easily scales to handle growing transaction volumes and datasets.
5. Enhanced Accuracy:
- Reduces false positives and improves fraud detection rates.
6. Regulatory Compliance Support:
- AI tools simplify audits and reporting, ensuring adherence to standards like GDPR, CCPA, and AML/KYC.
4. Challenges in AI and ML Implementation
1. Data Privacy and Security Concerns:
- Issue: Sensitive data exposure poses risks of breaches and non-compliance.
- Solution: Implement encryption, tokenization, and anonymization techniques.
2. Algorithm Bias:
- Issue: Biased training data can lead to inaccurate predictions.
- Solution: Use diverse datasets and regularly audit AI models.
3. Regulatory Uncertainty:
- Issue: AI's evolving nature complicates compliance frameworks.
- Solution: Work with RegTech providers to monitor regulatory changes.
4. Integration with Legacy Systems:
- Issue: Compatibility issues with older infrastructures.
- Solution: Deploy APIs and hybrid solutions for seamless integration.
5. Transparency and Explainability:
- Issue: Complex AI models can be difficult to interpret.
- Solution: Use Explainable AI (XAI) to provide transparency in decision-making.
5. Strategies for Implementing AI and ML in Risk Management
Step 1: Assess Risk Management Needs
- Identify vulnerabilities and determine where AI and ML can deliver value.
Step 2: Build Robust Data Infrastructure
- Invest in cloud platforms and big data tools for storage and analytics.
Step 3: Select AI and ML Tools
- Choose pre-built platforms or develop custom solutions tailored to fintech operations.
Step 4: Focus on Compliance and Security
- Ensure solutions comply with industry regulations and data protection laws.
Step 5: Train Models and Test Thoroughly
- Validate AI models with historical data before deployment.
Step 6: Monitor and Optimize Performance
- Continuously update models to adapt to new risks and patterns.
6. Future Trends in AI and ML for Risk Management
1. Predictive Risk Analytics:
- AI tools forecasting potential threats based on real-time data streams.
2. AI-Powered Behavioral Biometrics:
- Continuous monitoring of user behavior to detect anomalies and unauthorized access.
3. Automated Regulatory Compliance Systems:
- AI-driven solutions to generate reports and ensure real-time compliance.
4. Quantum Computing Integration:
- Advanced computation for faster risk modeling and encryption.
5. Decentralized AI Networks:
- Secure AI models leveraging blockchain for transparency and collaboration.
6. Explainable AI (XAI):
- Providing transparency in AI decisions to meet regulatory expectations.
AI and ML Are Redefining Risk Management in Fintech
AI and machine learning are transforming fintech risk management by offering scalable, proactive, and data-driven solutions. From fraud detection and AML compliance to cybersecurity and investment strategies, AI delivers unparalleled efficiency and accuracy.
However, fintech companies must address challenges like algorithm bias, privacy concerns, and regulatory uncertainties to maximize AI's potential. With careful implementation and continuous optimization, AI and ML will remain critical tools for managing risks in the evolving fintech landscape.
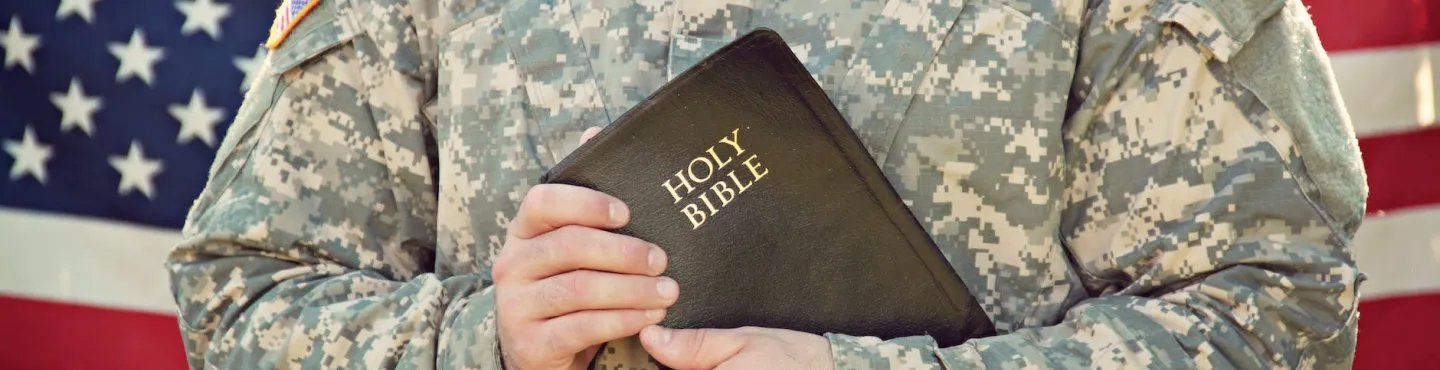